Learning to Use the Force: Fitting Repulsive Potentials in Density-Functional Tight-Binding with Gaussian Process Regression, Theoretical and Computational Chemistry, ChemRxiv
4.5 (421) In stock
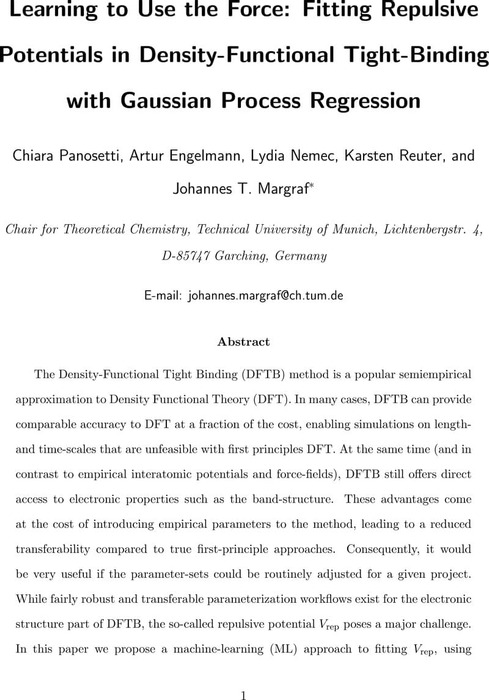
The Density-Functional Tight Binding (DFTB) method is a popular semiempirical approximation to Density Functional Theory (DFT). In many cases, DFTB can provide comparable accuracy to DFT at a fraction of the cost, enabling simulations on length- and time-scales that are unfeasible with first principles DFT. At the same time (and in contrast to empirical interatomic potentials and force-fields), DFTB still offers direct access to electronic properties such as the band-structure. These advantages come at the cost of introducing empirical parameters to the method, leading to a reduced transferability compared to true first-principle approaches. Consequently, it would be very useful if the parameter-sets could be routinely adjusted for a given project. While fairly robust and transferable parameterization workflows exist for the electronic structure part of DFTB, the so-called repulsive potential Vrep poses a major challenge. In this paper we propose a machine-learning (ML) approach to fitting Vrep, using Gaussian Process Regression (GPR). The use of GPR circumvents the need for non-linear or global parameter optimization, while at the same time offering arbitrary flexibility in terms of the functional form. We also show that the proposed method can be applied to multiple elements at once, by fitting repulsive potentials for organic molecules containing carbon, hydrogen and oxygen. Overall, the new approach removes focus from the choice of functional form and parameterization procedure, in favour of a data-driven philosophy.

Automated in Silico Design of Homogeneous Catalysts

PDF) Electrostatic Embedding of Machine Learning Potentials
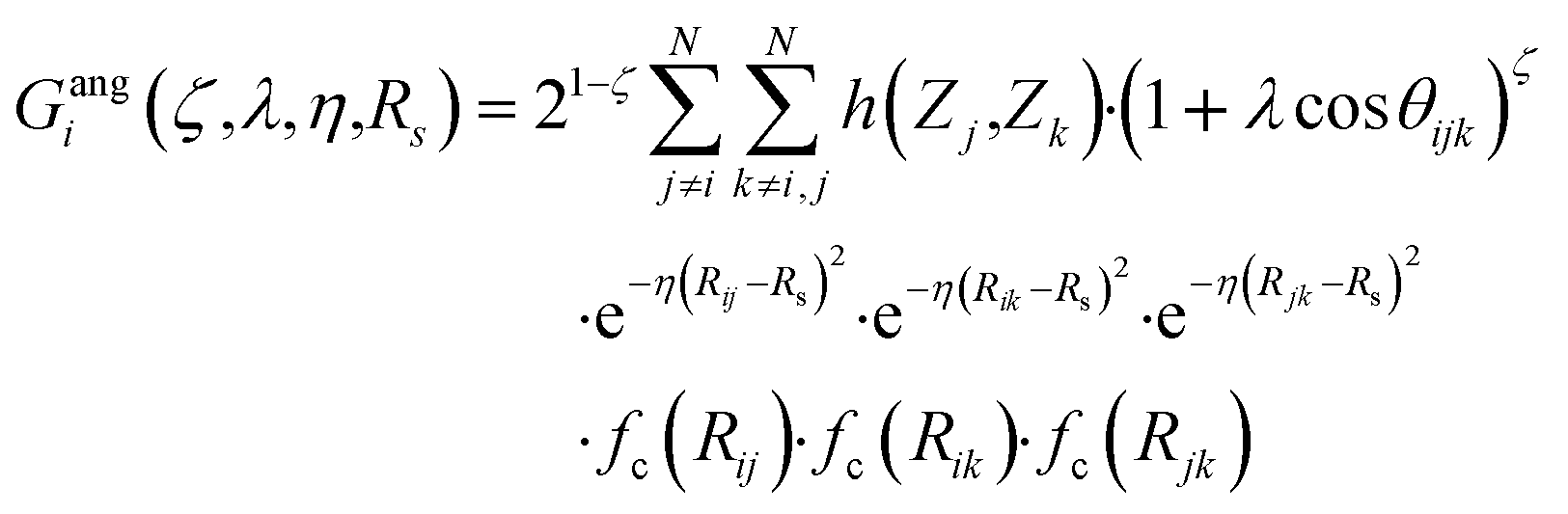
Neural network potentials for chemistry: concepts, applications

Interfacing q-AQUA with a Polarizable Force Field: The Best of
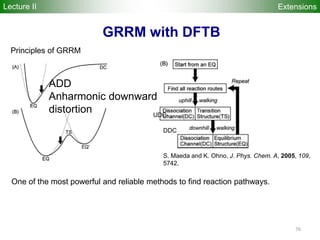
Density-Functional Tight-Binding (DFTB) as fast approximate DFT
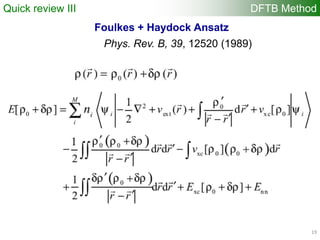
Density-Functional Tight-Binding (DFTB) as fast approximate DFT
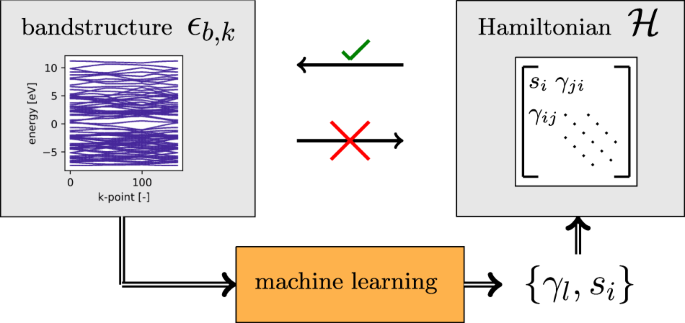
Machine learning sparse tight-binding parameters for defects
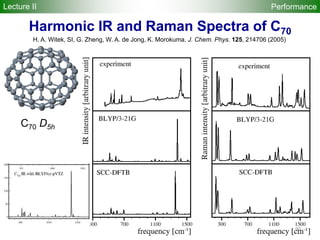
Density-Functional Tight-Binding (DFTB) as fast approximate DFT

PDF) Small basis set density-functional theory methods corrected
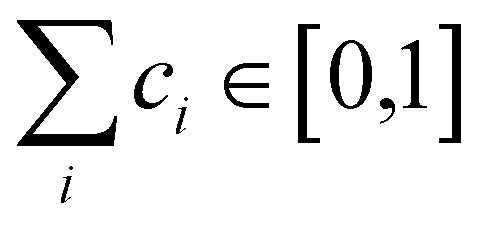
Neural network potentials for chemistry: concepts, applications

PDF) Semiempirical Hamiltonians learned from data can have
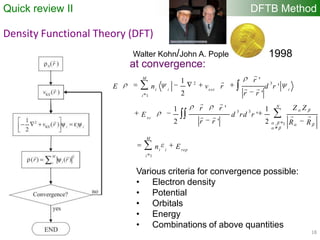
Density-Functional Tight-Binding (DFTB) as fast approximate DFT
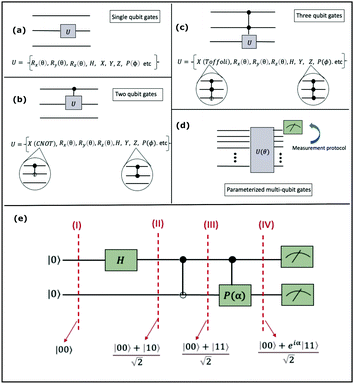
Quantum machine learning for chemistry and physics - Chemical Society Reviews (RSC Publishing) DOI:10.1039/D2CS00203E
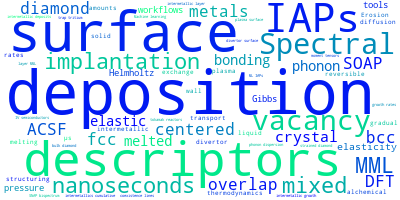
OpenKIM · SNAP ZuoChenLi 2019 Si MO_869330304805_000

Machine learning methods in photochemistry and photophysics - ScienceDirect
How To Keep Your Snowboard Bindings Tight
Turquoise Turtle knot - Step 8, tightening to finish (binding knot Stock Photo - Alamy
Tg 100 Manual Nylon Cable Tie Tightening Gun Cable Tie Tool - Temu Canada
- Nike Pro Combat 5-padded compression shorts size large Dri-fit
- Vestido Midi Zara Vestido Feminino Zara Nunca Usado 90407240
- Velcro One-Wrap® 8 x 3/4 Ties for Cables, Wires, and Cords
- Accessoires D'éclairage Pour Lampe De Table Ou Lampe De Sol Avec Abat-jour En Métal Ajouré, Noir, Dimensions De 7,48 Pouces X 5,9 Pouces X 5,11 Pouces
- Vuori Men's Strato Tech Hoodie Heather Grey
- Fab Five Archives – Sneaker History - Podcasts, Footwear News & Sneaker Culture